It is now undisputed that artificial intelligence with the right architecture and the right data-driven approaches will bring a quantum leap in the quality of document capture. When applied correctly, this also applies to line items. But why can’t every AI record individual positions equally well?
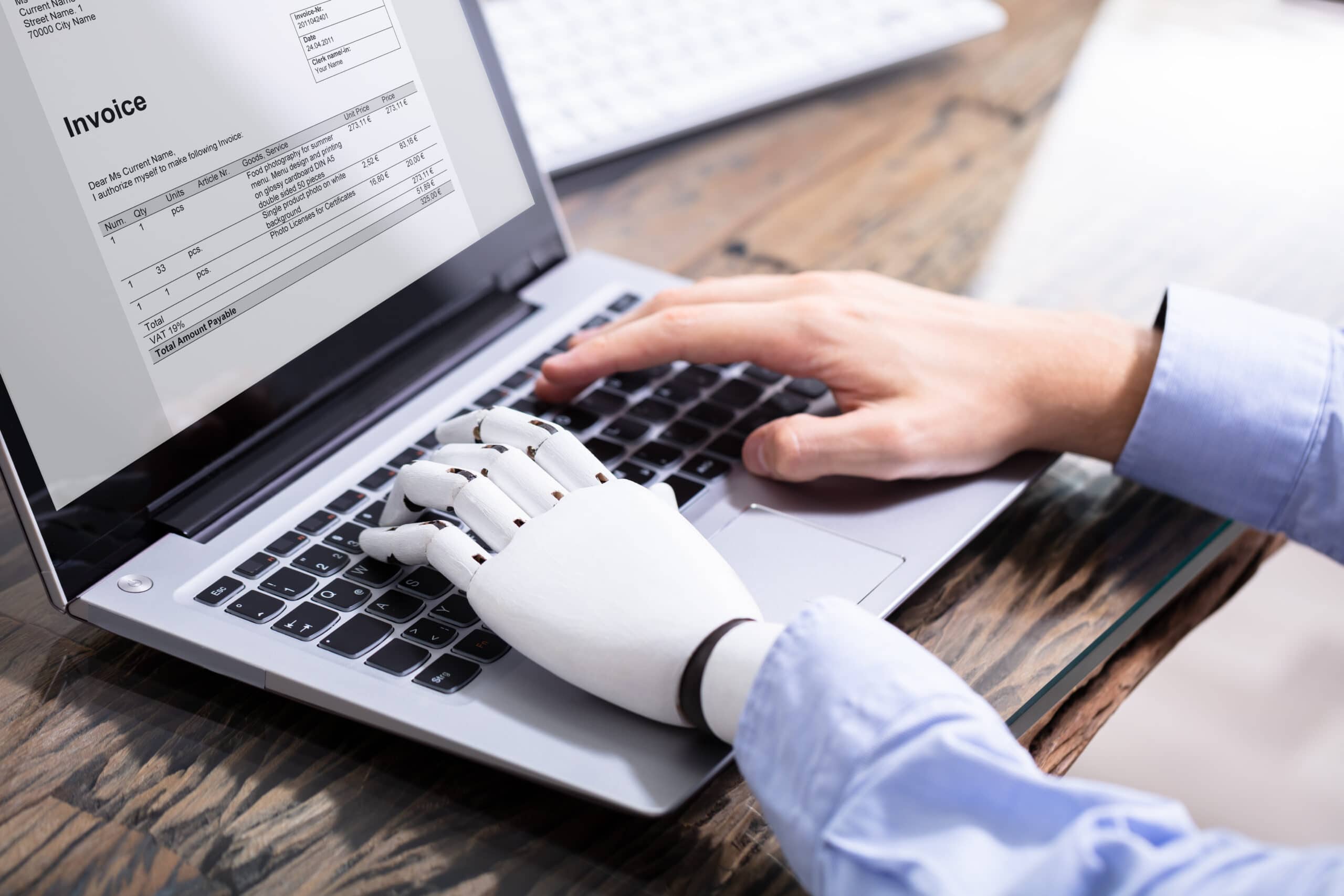
Is a Line Item Table a Table?
Rows, columns and the resulting cells should clarify semantic relationships in tables (see also Table definition).
From a legal point of view, invoices do not have any mandatory fields for individual items and there are no specific definitions for individual items neither. This leaves the authors with great lyrical and creative freedom, which is often fully exploited without regard to the reading habits of the recipients or their systems.
Further information on individual positions and text recognition is also available in this blog post: Invoice Recognition: Record Line Items with OCR
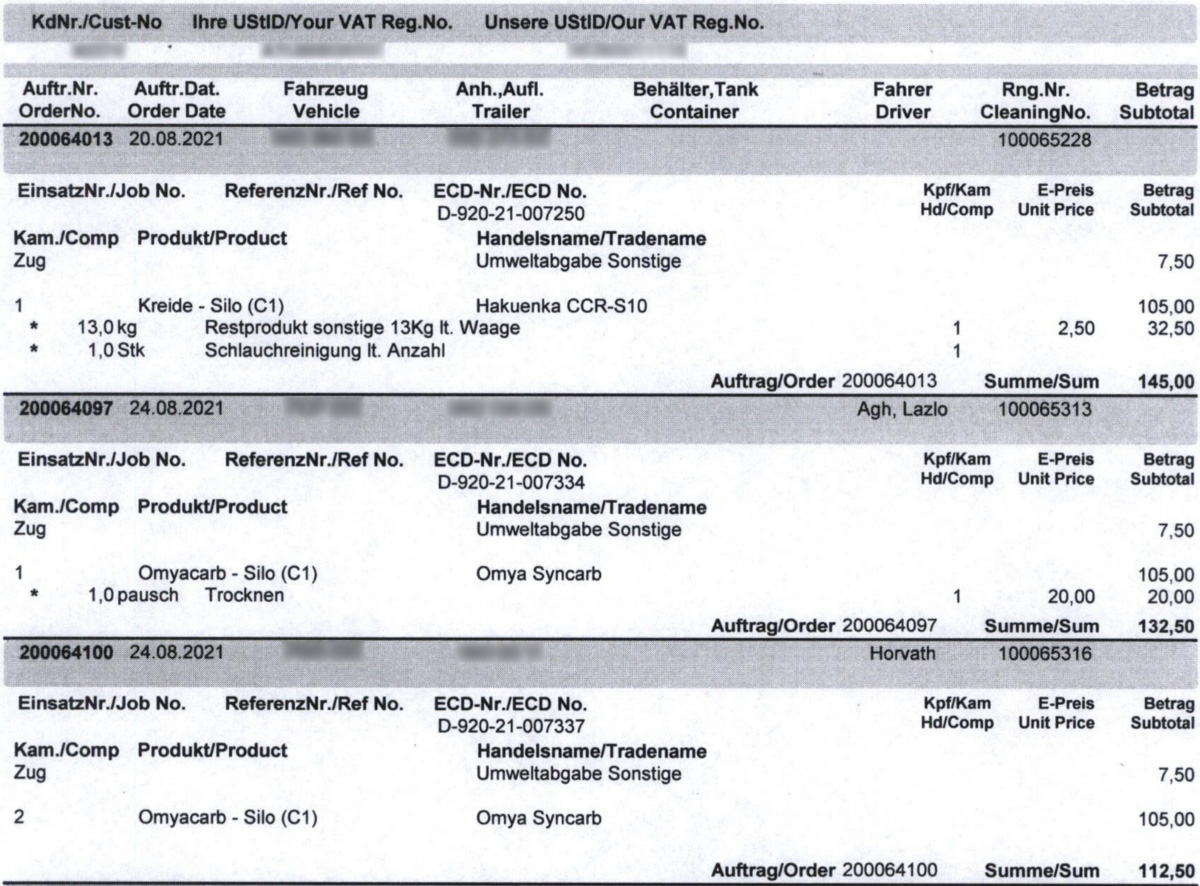
Which brings us to the biggest problem with entering line items. One speaks of tables or, in Denglish, of line item tables, although they are not tables in the traditional sense.
Memorizing vs. Understanding Line Items
Outdated, "smart" systems (i.e. systems with an origin before 2015) have a hard time here. These invoice entry systems use a so-called template approach. They learn by heart (with a certain tolerance) where characteristics of the individual items are on a specific invoice from a supplier. While this can still work for the header data of invoices and documents, these systems are overwhelmed when it comes to more complex line items. The structure of line items is more complex and less rigid compared to that of header data. You have to understand the structures and semantic relationships better here.
The right AI Architecture understands Invoices and Documents
In 2020, AI in the field of document capture experienced another boost. NLP and deep learning approaches from the field of computer vision were combined with each other, making it possible for the AI to learn semantic connections that were recognizable for humans. Based on these research results, it was now possible to further optimize the architectures and create new AI models that recognize "fuzzy" tables better. Furthermore, the "data-driven" approach was pushed. So the improvement of the models through targeted and continuous improvement of training data sets.
Training Data for Line Items: Chicken or Egg?
An AI learns based on data. In the simplest case, example data is provided as input with the associated solution (the desired output) of the AI for "practice". Of course, this is “easy” when there is historical sample data. In other words, companies that have manually entered masses of individual items in the past. However, since this is very time-consuming, most companies have either not recorded them at all or only recorded the fields that are absolutely necessary. Therefore, the mass data required for generalized models and deep learning is very limited on the market.
Training Data and Associated Training Value
Training data (or in our case, documents/invoices with the desired, associated acquisition data) represent a training value from the point of view of AI training (how much can the system learn from this document?).
We distinguish between extrinsic and intrinsic training values. A higher extrinsic training value helps, for example, to better learn the externally given, real problem. An example would be a previously completely unknown document layout that is highly relevant on the market or for customers.
An intrinsic training value comes from a model's weaknesses and is closely related to active learning. Put simply, this means that the model is not yet able to differentiate between certain situations, and that it builds up more "know-how" with the right examples.
Synthetic Line Items based on Real Documents
And this is exactly where the generation of synthetic data plays a key role. Synthetic data has no limitation on quantity, however the extrinsic and intrinsic training value must be high. At BLU DELTA we generate line items that correspond to the real situations and the model learns in an optimized way. In this way, we achieve significantly higher recognition rates than with traditional systems, both for special industry requirements and for our generalized AI model for line item detection.
If you would like to learn more about how line items are processed or how to improve their detection, please contact us. We look forward to every exchange.
BLU DELTA is a product for the automated capture of financial documents. Partners, but also finance departments, accounts payable accountants and tax advisors of our customers can use BLU DELTA to immediately relieve their employees of the time-consuming and mostly manual capture of documents by using BLU DELTA AI and Cloud.
BLU DELTA is an artificial intelligence from Blumatix Intelligence GmbH.